When Two Worlds Collide: AI and Observability Pipelines

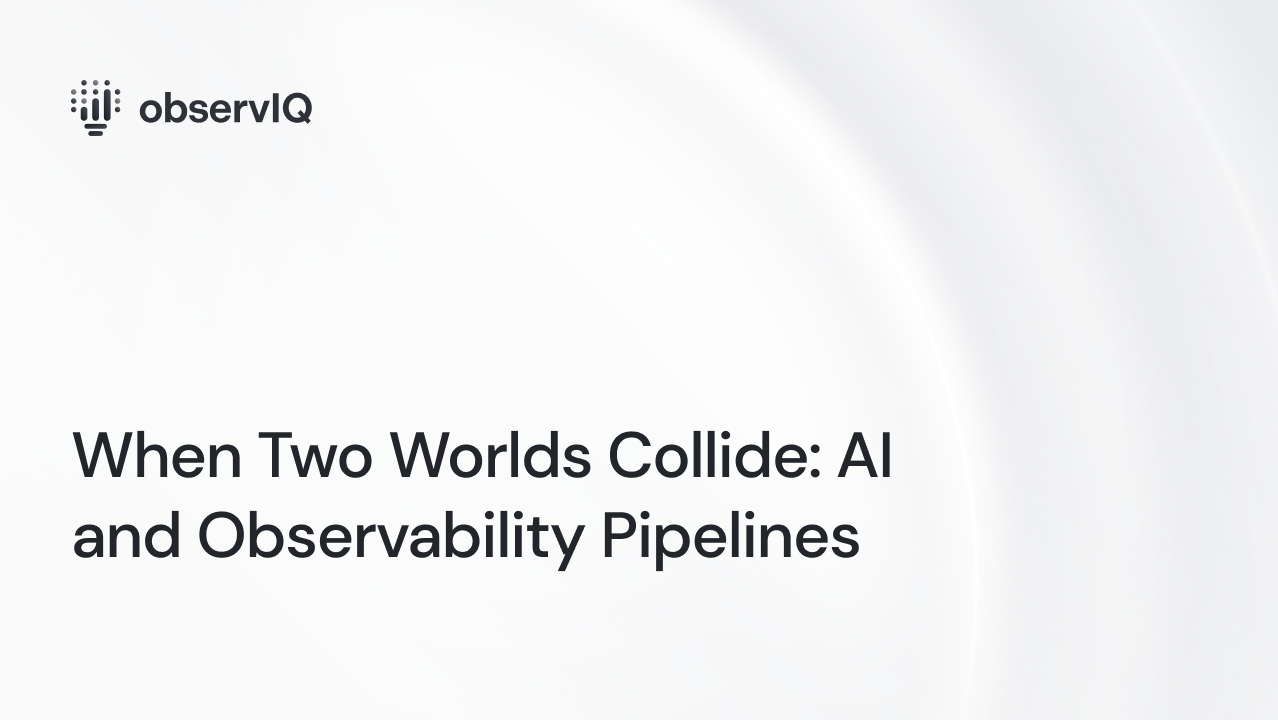
In today's data-driven world, ensuring the stability and efficiency of software applications is not just a need but a requirement. Enter observability. But as with any evolving technology, there's always room for growth. That growth, as it stands today, is the convergence of artificial intelligence (AI) with observability pipelines. In this blog, we'll explore the idea behind this merger and its potential.
Understanding Observability
As we stated in our previous “What is Observability” blog, our CEO, Mike Kelly, discussed observability, emphasizing its role in understanding a system's state with The Cube at Kubecon EU. Unlike monitoring, which targets specific metrics or events, observability offers a comprehensive view of a system's state, behavior, and performance. This understanding allows teams to proactively address issues, solve problems, and enhance system reliability.
Related Content: Splashing into Data Lakes: The Reservoir of Observability
The Need for AI in Observability
Telemetry data is growing exponentially and observability pipelines are now processing more data than ever before. According to a recent study by Gartner, “By 2026, 40% of log telemetry will be processed through a telemetry pipeline product, an increase from less than 10% in 2022”. However, even with robust visualization tools, spotting issues, trends, and anomalies in real time is becoming humanly impossible.
This is where the hot topic of Artificial Intelligence comes in as we get asked frequently, how can you incorporate AI into observability pipelines?
The merger of AI with observability is more than just an integration. It's about creating a symbiotic system (think yin yang symbol) where both technologies improve each other's capabilities. Here are some examples:
- Data Processing: Observability pipeline tools, such as BindPlane OP, can preprocess data, structuring it in ways optimal for AI models. This can involve standardizing data, filtering out noise, and deriving additional features.
- Feedback Loops: AI models improve with feedback. Observability pipelines can continuously feedback anomalies that were actual issues, allowing models to refine their detection capabilities. It’s like having your own personal detective, helping reduce the time spent figuring out what went wrong.
- Automated Responses: Based on AI's real-time analysis, observability pipelines can automate certain corrective actions, while also giving suggestions for next steps such as filtering for further data reduction.
- End-to-End Insights: With AI analyzing data throughout the pipeline, organizations can get easy-to-understand insights that span from infrastructure health to user behavior analytics. This helps make our systems better and ultimately aids in user satisfaction.
Even though AI is the popular buzzword, the integration of AI and observability is still in its infancy but is gaining traction. The two will form an even tighter bond as AI models become more sophisticated and observability data becomes richer. This evolution will help create more efficient and intelligent systems, enabling organizations to gain a great wealth of insights and stability as they continue their own growth and gain trust in artificial intelligence’s accuracy.
